Angélique Moon, Director of Product Management for Consumer Goods at Oracle, briefed me recently on Oracle’s Demand Signal Repository (DSR) solution. At the end of the presentation, Ms. Moon and I bemoaned the fact that the vocabulary used to describe these types of products is not very good. Shortly after, I had a similar conversation with Razat Guarav, the SVP of Sales at i2 Technologies (both Oracle and i2 are ARC clients). For me, this topic goes beyond semantics. If the products and applications in this area are not properly named, then many supply chain professionals will not understand what they do. And what they do is the next frontier of value chain management in the consumer goods value chain.
Ms. Moon made the point that the term “demand signal” focuses you on the demand data you are using. The term “demand sensing” is similar in that regard. However, for most companies, the most important demand signal they sense (i.e., demand data they consume) is point-of-sale (POS) data. Almost all consumer goods supply chains make use of demand signals; unfortunately, most of them use shipments from their distribution centers (DCs) to retailer DCs as their demand signal. We’ve known for years that using shipment data for your demand forecasts leads to the bullwhip effect.
From a demand data perspective, if we used the term “store consumption” data, most supply chain folks would interpret this as POS data. However, the folks in marketing and retail sales accounts, who also need to use the data in a DSR, would likely confuse this with syndicated data. They would reserve the term “POS” exclusively for data received directly from a retailer. A term like “downstream data” could provide a clearer meaning to all. At this point, however, the term “Demand Signal Repository” is well established, so I don’t see it changing to “Downstream Data Repository” even though I think it’s less confusing.
A DSR holds downstream data, but it is the applications and analytics that reside on top of the DSR that provide better plans or responsiveness. Several DSR vendors have made the point to me that by feeding DSR data into the right kind of supply chain application, your processes become “demand driven.” So the term “demand driven” refers to the nature of a supply chain process, while DSR refers to the data.
But when most supply chain professionals hear the term “demand driven,” they think of a fast and responsive supply chain. DSR supply chain applications will improve your supply chain, but they won’t necessarily make your supply chain fast and responsive. What a company accomplishes with granular store-level demand data depends upon what type of supply chain it has and what it is trying to accomplish.
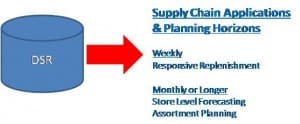
A food and beverage manufacturer, for example, can use DSR data (POS, store inventories, shipments from retail DCs) to become more demand driven by making its replenishment process more responsive to what is actually selling in a grocery chain’s stores.
But if you are an apparel manufacturer with a supply chain that starts in China supplying retail outlets in North America or Europe, DSR data will not make your supply chain “demand driven” in the sense that it becomes more highly responsive. Lead times of three to six months will make that impossible. But DSR data (POS, store loyalty, syndicated data, etc.) will improve an apparel company’s demand forecasts. Thus, instead of speaking of “demand driven” applications, we should really speak of supply chain applications that are capable of leveraging downstream data.
Some supply chain professionals speak of “sell into” versus “sell through.” The former applies to shipments to a retailer’s DC, while “sell through” refers to when consumers take product off the shelf and but it. DSR applications, therefore, can be considered “sell through” applications. The downside of this term is that it sounds too much like a Customer Relationship Management (CRM) application.
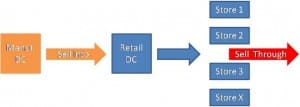
Mr. Guarav refers to i2’s solutions in this area as being focused on “shelf-centered collaboration.” This is a term I like because a core part of the emerging DSR-based predictive analytics are focused on reducing out-of-stocks, detecting phantom store inventory, and discovering the root causes of both. To the extent that these analytics have workflows attached to them, for example, having merchandisers check a particular store for phantom inventory, these could also be considered “shelf centered applications,” which is the best terminology I’ve heard in this area.
Leave a Reply