On April 22nd RedPrairie issued a press release announcing that it was adding “flowcasting” to its product suite. Despite reading the press release carefully, I couldn’t fully grasp what “flowcasting” was. Fortunately, RedPrairie’s management team visited us the next day and we discussed this new solution.
It turns out that “flowcasting” is synonymous with “demand sensing” and “sense and respond”. The industry has not settled yet on a single term to describe this emerging class of solutions, but I will use “demand sensing” because it is used more frequently.
What is the difference between demand sensing and traditional demand management? In traditional demand management, you start by summing historical sales and shipment data across all customers over some time period. This historical demand allows you to make baseline revenue and unit forecasts. The forecast is done at different levels of aggregation. Typically, the most granular forecast is by SKU by the manufacturer’s distribution centers (DCs). Usually, several DCs are supplied by a particular factory, and there is also a factory-level forecast. If several factories can manufacture a SKU, there would probably also be a regional or worldwide forecast. So while we talk about “forecast” in the singular, this is only true at the highest level where we have summed the forecast across all products and factories for some period of time. That global forecast is disaggregated into multiple “forecasts” for particular factories, DCs, SKUs.
The forecast is then shared with sales and marketing, and possibly customers, and is adjusted based on upcoming promotions, new product introductions, and so forth. While the forecast is used by various groups in the company, the primary driver for the forecast is to create manufacturing plans, often on a monthly schedule (e.g., SKU by factory by month). At many manufacturing companies, the production plan, ideally in combination with DC-level forecasts, then drives replenishment planning for deliveries from a factory to a DC.
In contrast, demand sensing is based on more granular demand data. In the case of RedPrairie’s solution, demand data is based on a customer’s historical POS and in-store inventory data. Then, as is the case for traditional demand management, users collaborate to adjust the forecast for promotions and new product introductions. And the primary purpose of this forecast is not manufacturing planning, but to drive better replenishment planning between the factory and the DCs.
While there are some differences in how individual manufacturers go about their forecasting process, and in particular Demand Management and Sensing solutions, in general the table below highlights the differences as I see them.
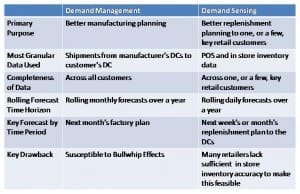
RedPrairie showed us the results achieved by a very large Food manufacturer in supplying one of its largest retail customers. This food company was having problems creating accurate replenishment plans, particularly around the holidays. In 2008, as compared to 2007, their holiday period store sales increased by 20 percent, DC out-of-stocks decreased by 86 percent, and DC Inventory turns increased by 10 percent.
While it’s nice to hear this success story from RedPrairie, I’d prefer to hear it directly from the food manufacturer. RedPrairie will be presenting its new flowcasting product next week at its upcoming user conference and they are hopeful a supply chain executive from the food company will co-present with them. My colleague Adrian Gonzalez is attending the conference (he’s speaking on “Is Green Good for Business During a Recession?”), so if this food manufacturer presents more details about their success with flowcasting, you’ll hear about it here first.
Leave a Reply